This concept certainly isn’t new. Routine equipment checks and preventive maintenance in general have been the responsibility of every maintenance department for decades. But here’s the good part.
You can use AI and Internet of Things (IoT) sensors to go beyond preventive maintenance to implement predictive maintenance.
Preventive maintenance prevents failures with inspections and services performed at predetermined intervals. Predictive maintenance uses large volumes of data and advanced analytics to anticipate the likelihood of failure based on the history and current status of a specific piece of equipment and recommends service before it happens.
How do you like the sound of that? It’s the sound of asset performance optimization. This figure traces the evolution of this concept.

Spying on Your Machines
Asset performance optimization (APO) collects the digital output from IoT-enabled equipment and associated processes, analyzes the data, tracks performance, and makes recommendations regarding maintenance. APO allows you to forecast future needs and perform predictive maintenance before immediate actions are needed.Although some machines run continuously with little need for maintenance, others require much more care and attention to operate at their best level. Determining which equipment needs more frequent servicing can be time-consuming and tedious. Often maintenance guidelines rely heavily on guesswork. Time frames for tune-ups tend to be little more than suggestions, based on information such as shop manuals and a recommendation from the lead mechanics rather than hard data, such as metrics from the performance history of each piece of equipment, including downtime and previous failures.
APO, on the other hand, analyzes both structured and unstructured data, such as field notes, to add context for equipment readings and deliver more precise recommendations. Using IoT devices, APO systems gather data from sensors, EIM systems, and external sources. It then uses AI to acquire, merge, manage, and analyze this information, presenting the results using real-time dashboards that can be shared and reviewed quickly for at-a-glance updates. Throughout the lifespan of the equipment, through regular use and maintenance, the system continues to learn and improve its insights over time.
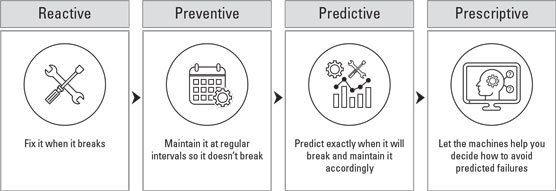
Fixing It Before It Breaks
APO allows you to take a strategic approach to predictive maintenance by focusing on what will make the greatest difference to your operation. Unforeseen equipment malfunctions and downtime cause disruptions, which can ultimately jeopardize project timelines, customer satisfaction, and business revenue.These are benefits of APO:
- Smoother, more predictable operations: Equipment issues are addressed preemptively instead of after a disruption occurs, leading to greater overall operational efficiency. Implementing APO can help companies achieve a 70-percent elimination of unanticipated equipment failure.
- Reduced downtime: Preventive maintenance typically reduces machine downtime by 30 to 50 percent.
- Boosted productivity: In addition to reducing downtime, predictive maintenance allows you to become more strategic in scheduling maintenance. This also can uncover any routine maintenance tasks that can be performed at less frequent intervals.
- Lowered costs: APO can reduce the time required to plan maintenance by 20 to 50 percent, increase equipment uptime and availability by 10 to 20 percent, and reduce overall maintenance costs by 5 to 10 percent, according to a Deloitte study.
- Increased customer satisfaction: Assets nearing failures can sacrifice production quality, cause service outages, and create other circumstances that ultimately affect the customer. By preventing these issues from happening, APO helps companies achieve and maintain better customer satisfaction.
- Improved safety outcomes: Equipment malfunctions can result in serious injury, but often companies don’t know a system is about to fail until it’s too late. PwC reports that APO and predictive maintenance can improve maintenance safety by up to 14 percent.
- Reduced risk of litigation and penalties: With fewer breakdowns and disruptions to service, organizations can minimize their risk of costly fines, lawsuits, and subsequent reputational damage.
Learning from the Future
APO solutions allow you to enhance your operations by making your machines smarter and sharing that intelligence with your workforce, thereby maximizing the value of both your human teams and your mechanical equipment. As the age of AI advances, the companies that thrive will be those that find the best ways to harness data for improved operational performance.Data collection
APO continuously collects data on mechanical performance from IoT sensors in virtually any type of device or machine, ranging from hydraulic brake system sensors on a train to temperature monitors inside industrial and medical refrigerators holding sensitive products. The system collects numerous data points from the sensors, blending them with other sources, and analyzes the results.For example, in the case of a hydraulic brake system, APO compares current data to historical performance records, including failure reports, to deliver predictive maintenance insights. When further data inputs are blended with this specific brake data, even richer and more accurate recommendations can be delivered.
Additional input samples from internal and third-party sources can be blended to provide context and greater insight; these types of input can be invaluable:
- Weather data
- Maintenance recommendations from manuals
- Supplier quality data
- Historical brake maintenance schedules and failure rates
- Passenger travel analysis
- Heavy or unusual usage data
Using this comprehensive blend of data, you can implement an APO solution to recognize patterns and perform an in-depth analysis in multiple applications, from manufacturing plants to utilities and even healthcare. The data can include metrics such as temperature, movement, light exposure, and more collected from IoT sensors on fleets, plants, pipelines, medical imaging equipment, jets, grids, and any other Internet-enabled device.
Analysis
AI uses big data analytics and natural-language processing to derive key data points from structured data as well as unstructured content such as field notes and equipment manuals.You can use AI to analyze this information and relevant historical data to identify patterns and generate questions that help engineers, maintenance supervisors, production managers, and other key personnel make informed and timely decisions. APO solutions use these patterns to make predictive conclusions that help you answer questions in various areas, such as these:
- Timing: Am I performing inspections at appropriate intervals? Would shortening the intervals improve overall uptime? Or could I afford to lengthen the intervals to reduce resource expenditure?
- Quality: Could defective components be slipping through my inspections and leading to downtime? If so, how can I improve the inspection process to prevent this issue moving forward?
- Design: Can my design be modified to reduce future failures?