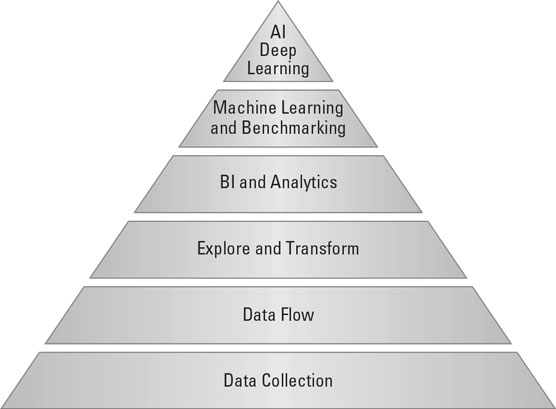
Data collection
Data collection is the foundation of the pyramid, the stage where you identify what data you need and what is available. If the goal is a user-facing product, are all relevant interactions logged? If it is a sensor, what data is coming through and how? Without data, no machine learning or AI solution can learn or predict outcomes.Data flow
Identify how the data flows through the system. Is there a reliable stream or extract, transform, and load (ETL) process established? Where is the data stored, and how easy is it to access and analyze?Explore and transform
This is a time-consuming and underestimated stage of the data science project life cycle. At this point, you realize you are missing data, your machine sensors are unreliable, or you are not tracking relevant information about customers. You may be forced to return to data collection and ensure the foundation is solid before moving forward.Business intelligence and analytics
After you can reliably explore and clean data, you can start building what is traditionally thought of as business intelligence or analytics, such as defining key metrics to track, identifying how seasonality impacts product sales and operations, segmenting users based on demographic factors, and the like.Now is the time to determine:
- The features or attributes to include in machine learning models
- The training data the machine will need to learn
- What you want to predict and automate
- How to create the labels from which the machine will learn
You can create labels automatically, such as the system logging a machine event in the back-end system, or through a manual process, such as when an engineer reports an issue during a routine inspection and the result is manually added to the data.