In many cases, seasonal patterns are removed from time-series data when they’re released on public databases. Data that has been stripped of its seasonal patterns is referred to as seasonally adjusted or deseasonalized data.
In order to obtain a goodness-of-fit measure that isolates the influence of your independent variables, you must estimate your model with deseasonalized values for both your dependent and independent variables. Here’s how to do just that:
Regress your dependent variable on the seasonal dummy variables to obtain the estimated function
and retain the residuals from this regression.
Regress each of your independent variables on the seasonal dummy variables to obtain the estimated functions
where k represents a specific independent variable, and retain the residuals from all k of these regressions.
Regress the residuals obtained in Step 1
on the residuals obtained in Step 2
to estimate
The R-squared from this regression provides a better measure of fit when the time series exhibits considerable seasonality.
The traditional R-squared can be overinflated when the data contains significant seasonal patterns. If you encounter this situation, simply estimate a regression with deseasonalized data to find an alternative R-squared value.
The figure uses STATA to estimate the impact of log monthly unemployment and a time trend on the log of souvenir sales between 1987 and 1993. First, the model is estimated with the raw data, and then the model is estimated with deseasonalized data.
The output for the intermediate steps is excluded to save space. As expected, the R-squared is smaller after the data is deseasonalized (0.9106 compared to 0.9539), but the difference isn’t big. The coefficient estimates for the unemployment and trend variables are similar in both regressions, so the results imply that the role of the independent variables isn’t affected by seasonal patterns.
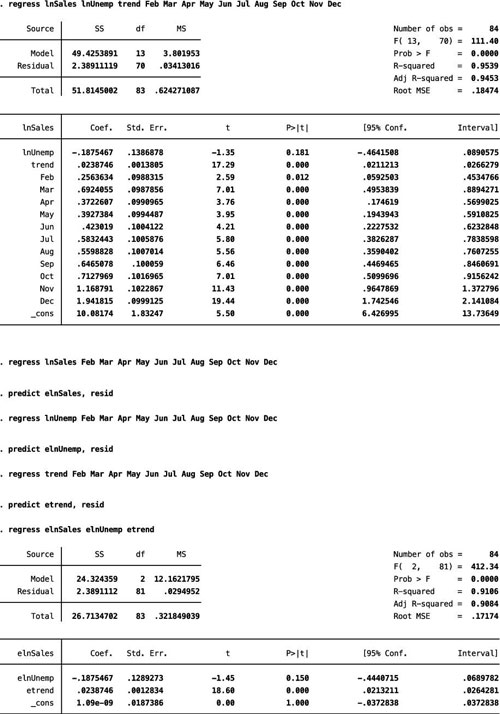
Econometricians mainly estimate the regression model with deseasonalized data to derive the explanatory power of the other independent variables. Your primary econometric results, however, should report the estimates from the model with the raw data and season dummy variables.