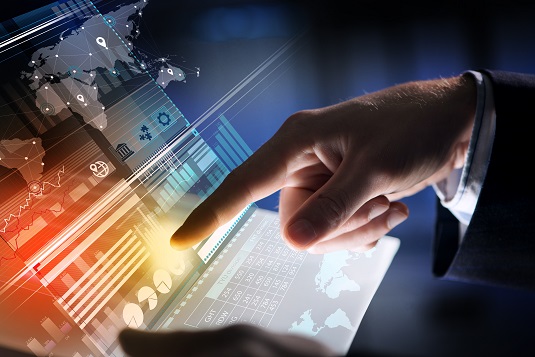
Don't tolerate top management's ignorance of data science
A fundamental misunderstanding occurs in the area of data science regarding the target group for data science training. The common view is that as long as the skill set for the data scientists themselves is improved, or for the software engineers who are training to become data scientists, you are spot-on. However, by adopting that approach, the company runs the significant risk of alienating the data science team from the rest of the organization. Managers and leaders are often forgotten.If managers don’t understand or trust the work done by the data scientists, the outcome won’t be utilized in the organization and insights won’t be put into action. So, the main question to ask is how to secure full utilization of the data science investment if the results cannot be interpreted by management.
This is one of the most common mistakes committed by companies today, and the fact is that there’s also little training and coaching available for line management and for leaders. But without some level of understanding of data science at the management level, how can the right strategy be put in place, and how can you expect management to dare to use the statistical results to make substantive decisions?Without management understanding of data science, it’s not only difficult to capture the full business opportunity for the company, but it might also lead to further alienation of the data science team or to termination of the team altogether.
Don't believe that AI is magic
Data science is all about data, statistics, and algorithms. There’s nothing magic about it — the machine does what it’s told to do. However, the notion that the machine can learn causes some to think that it has the full ability to learn by itself. To some extent, that is correct — the machine can learn — but it's correct only within the boundaries you set up for it. (No magic, in other words!) A machine cannot solve problems by itself, unless a machine is allowed to develop such a design. But that’s advanced technology and not today’s reality.Overestimating what artificial intelligence can do for your company can really set you off on the wrong track, building up expectations that can never be met. This could lead to severe consequences both within the company and externally, with impacts not just in terms of trust and reliability but also in terms of financial performance. As important as it is not to underestimate the potential in artificial intelligence, one should also avoid the opposite extreme, where its potential is overestimated.
Let’s repeat: Artificial intelligence isn’t magic. Yes, it’s called artificial intelligence, but a more correct definition is actually algorithmic intelligence. Why? Because at the end of the day, very advanced mathematics are applied to huge amounts of data, with the ability to dynamically interact with a defined environment in real-time.Don't approach data science as a race to the death between man and machine
Some people tend to believe that task automation, driven by machine learning predictions, truly means the end of humans in the workplace. That prediction isn’t one that everyone believes in. However, the presence of AI does mean a significant change in competence and skill sets as well as a change in which job roles will be relevant and which types of responsibilities will be the focus in the workplace.Like the introduction of the Internet in the workplace, introducing artificial intelligence in a more mainstream format will change what jobs are and how they’re performed. There will be a lot less “hands-on” work, even in the software business. And yes, machines will most probably do a lot of the basic software development going forward, which means that people in the hardware-related industry will not be the only ones replaced. At the end of the day, basically all humans will be impacted as machine learning/artificial intelligence and automation capabilities and capacity expand and evolve beyond what is possible to do today.
However, this also means that humans can move on to perform other tasks that are different from the ones we do today — managing and monitoring models and algorithms and their performance, for example, or setting priorities and acting as a human fallback solution in cooperation with the machine. Other typical human tasks might be managing legal concerns related to data, evaluating ethical aspects of algorithm-based decision-making, or driving standardization in data science. You could say that the new human tasks will be focused on managing the machines that manage the original tasks — tasks that were previously perceived to be either boring and repetitive or too complex to execute at all.
This "putting man against machine" business isn’t the way to approach your data science implementation. Allowing the narrative to be framed that way may scare your employees and even prompt them to leave the company, which isn’t what you want. Your employees are valuable assets that you need in the next stages as well, but perhaps in new roles and with new acquired skill sets.
Embrace what the machine learning/artificial intelligence technology can do for a specific line of business. Company leaders who understand how to utilize these techniques in a balanced approach between man and machine to augment the total performance and let the company evolve beyond its current business are the leaders whose companies will succeed.
Don't underestimate the potential of AI
As strange as it may seem, some companies just don’t understand how transformative artificial intelligence really is. They refuse to see the fundamental shift that is already starting to transform society, and cannot see artificial intelligence as anything other than just another software technique or a set of new programming languages.The key here is to a) take the time to truly understand what data science is really all about and to b) not be afraid to accept help from experts to identify and explain the strategic potential for your specific business. Because the area of data science is complex, it requires domain expertise and experience in terms of both the development of a strategy and its implementation. It also requires the ability to read and interpret where the market is moving in this area.
By underestimating the impact that artificial intelligence can have on your business, you run the risk of significantly limiting the future expansion of your company. Later, once the true potential is really understood, you will find yourself entering the game too late and being equipped with the wrong skill set. You may finally be put out of business by competitors that had seen the potential much earlier and therefore invested earlier and smarter in artificial intelligence.
Don't underestimate the needed data science skill set
A typical sign of companies underinvesting in data science is when you find small, isolated islands of data science competence spread out in different parts of a large company. In smaller companies, you see a similar symptom when a small-but-competent data science team is working on the most important project in the company but the only one outside the team that realizes its importance is an outsider like yourself.Both of these examples are signs that top management in the company has not understood the potential of data science. They have simply realized that something is happening in this area in the market and are just following a trend to make sure that data science doesn’t pass them by.
If the awareness and competency level of management doesn’t improve, the area will continue to be underinvested, distributed in a way that it cannot reach critical mass, and therefore rendered incapable of being scaled up at a later stage.
Don't think that a dashboard is the end objective of data science
It may sound strange for, someone knowledgeable in data science, to say that anyone can think that the main outcome of data science is a dashboard. Rest assured, however, that this is a common misunderstanding. This isn’t only wrong — it’s also one of the main reasons that many companies fail with their data science investment.At many companies, management tends to think that the main purpose of analytics and artificial intelligence is to use all that big data that has been pumped into the expensive data lake, to automate tasks and report on progress. Given such a mindset, it should come as no surprise that the main focus of management would be to use these techniques to answer their questions with statistically proven methods that could produce results that could be visualized in a nice-looking dashboard. For someone new to the field of data science, that might actually seem like a good approach. Unfortunately, they would be wrong.
To be absolutely clear, the main objective of analytics and machine learning/artificial intelligence isn’t simply to do what you've always done but using more machines. The idea is to be able to move beyond what you’re able to do today and tackle new frontiers.
If the only end goal was to create a dashboard in order to answer some questions posed by a manager, there would be no need to create a data-driven organization. The idea here is that, in a data-driven organization, it all starts with the data and not with the manager and the dashboard. The starting point is what the data is indicating that you need to look at, analyze, understand, and act on. Analysis should be predictive, in order for the organization to be proactive and for its actions to be preventive.The role of the dashboard should be to surprise you with new insights and make you discover new questions you should be asking — not to answer the questions you've already come up with. It should enable teams to monitor and learn from ongoing preventive actions. The dashboard should also support human or machine discovery of potential trends and forecasts in order to make long-term strategic decisions.
In the real world, the steps needed to design a dashboard tend to end up being the most important tasks to discuss and focus on. Often, dashboards end up driving everything that is done in the data science implementation program, totally missing the point about keeping an open and exploratory approach to the data. This tends to happen because the dashboard is the simplest and most concrete deliverable to understand and hold on to in this new, complex, and constantly changing environment. In this sense, it acts like a crutch for those unwilling or unable to grasp the full potential of a data-driven business.
You run the great risk of missing the whole point of being data driven when your starting point is all about designing the dashboard and laying down all the questions from the start. By doing so, you assume that you already know which questions are important. But how can you be sure of that? In a society and a market now undergoing huge transformations, if you don’t look at the data first and let the algorithms do the work of finding the patterns and deviations hiding there, you might end up looking at the entirely wrong problem for your business.
Don't forget about the ethical aspects of AI
What does artificial intelligence ethics actually refer to, and why do you think it’s of the utmost importance? Well, there are many aspects surrounding the idea of ethics in AI, many of which can have a severe impact on the artificial intelligence results. One obvious but important ethical consideration is the need to avoid machine bias in the algorithms — biases where human preconceptions of race, gender, class, or other discriminatory aspects are unconsciously built into the models and algorithms.Usually, people tend to believe that they don’t have biased opinions, but the truth is that everyone has them, more or less. People tend to lean in one direction, subconsciously or not. Modeling that tendency into self-learning algorithms can have severe consequences on the performance of the company´s algorithms.
One example that comes to mind involves an innovative, online, and artificial-intelligence-driven beauty contest. The algorithm had learned to search for the ten most beautiful women in the US, using only digital photos of women. But when studying the result from the contest, it became clear that something must have gone wrong: All of the ten most beautiful women selected by the algorithm were white, blonde, and blue-eyed. So, when studying the algorithm again, it turned out that the training set used for the algorithm had a majority of white, blonde, and blue-eyed women in it, which taught the machine that this was the desired look.
Other aspects in addition to machine bias include areas such as the use of personal information, the reproducibility of results outside the lab environment, and the explainability of AI insights or decisions. It’s also worth noting that this last aspect is now a law within the GDPR (General Data Protection Regulation) in the EU.
Ethical considerations are for our own, human protection as machine intelligence evolves over time. You must think about such aspects early on. It’s not only a fundamental aspect to consider as part of your data science investment, but it’s actually also hugely important to consider already from the start, when designing your business models, architecture, infrastructure, ways of working, and the teams themselves. Not wanting to break the law is of course important, but securing a sustainable and trustworthy evolution of artificial intelligence in your business is far more important.
Don't forget to consider the legal rights to the data
When becoming data driven, one of the most common mistakes is to forget to make a proper analysis of which data is needed. Even if your main ambition with your data science investment is focused on internal efficiency and data-driven operations, this is still a fundamental area to address.Once the data need is analyzed, it’s not unusual to discover that you need other types of data than you originally thought. It might be data other than just internally generated data, owned by you. An example might be faults found in your products or services, or perhaps performance related data. It could even be the more sensitive type of data, which falls under the category of privacy data, related to how your products or services are being used by your customers.
Data privacy is an area that’s getting more and more attention, in society with consumers’ enhanced awareness of how their data is being used and also in terms of new laws and regulations on data. One concrete example is the General Data Protection and Regulation law (GDPR), introduced in 2018 within the EU with significant penalties for violators.
Although you might not have any plans for monetizing your data or to build new products based on the data, the whole rights issue is still central — even when all you want to do is analyze the data in order to better understand your business, enhance and innovate the current portfolio, or just improve the efficiency of your operations.
No matter what your reasons are for using the data, you still need legal rights in place in order to use it! It’s absolutely vital to address this early on as part of the development of your data strategy. If you don’t, you might end up either violating the law regulating data usage and ownership or being stuck in terms of not being able to sell your new fantastic product or service because it’s using data you aren’t entitled to use.
Don't ignore the scale of change needed
If you don't take the time to properly sketch out the different change scenarios for your business when introducing a data science strategy, you most likely will fail. The fundamental shift needed in the company to become truly data, analytics, and machine driven is significant and should not be underestimated.The most common mistakes in data science related to managing change are listed here:
- Underestimating the scope of the change and not taking seriously enough what has to happen
- Failing to recognize that business models are sure to be impacted when introducing data science
- Approaching customers with a value argumentation based on introducing data science techniques without explicitly explaining what the customer value is
- Pricing models to stay the same or not reflect the increased value, only the lowered cost
- Focusing single-mindedly on cost efficiency when it comes to business operational changes
- Neither measuring nor understanding operational improvements
- Carrying out organizational changes on so small a scale that everything stays the same in practice, ensuring that the actual change never occurs
- Building the cost and dimensioning model on old and outdated criteria, therefore ensuring that the model won’t capture the new values
- Failing to see the change that data science imposes on the company and not understanding that change from an ecosystem perspective
- Underestimating the need for communication related to the change
Don't forget the measurements needed to prove the value of data science
A common mistake is to forget to introduce baseline measurements before the data science investment is made and implemented. Most of the focus in these cases tends to be on the future measurements and the results targeted with the investment. This is usually because of a resistance toward investing in new measurements in the current situation, because it’s being abandoned for the new strategy. Unfortunately, this means that the company will lack the ability to statistically prove the value of the investment in the next step. Don’t fall into that trap! It could truly backfire on the entire strategic ambition, when top management or even the board of directors asks what the value was of this major investment.Financially, you could, of course, be able to motivate the investment on a high level; however, it would be difficult to prove individual parts. Efficiency gains such as speed, agility, automation level, and process reactiveness versus proactiveness are values that are more difficult to prove and put a number on if you haven’t secured a measurement baseline before executing your data science strategy.