To describe the scope of a CDO, you first need to determine how the position relates to that of a chief analytics officer (CAO). Although the CDO and the CAO are two distinct roles, these two positions are customarily held by the same person or else only one role, the CDO role, is used, but when these roles are combined into one, it is sometimes also referred to as a CDAO role. However, in situations where these two roles are separate and held by two different functions, the main difference can be summarized by the title itself: data versus analytics. The main difference in the area of responsibility is captured below.
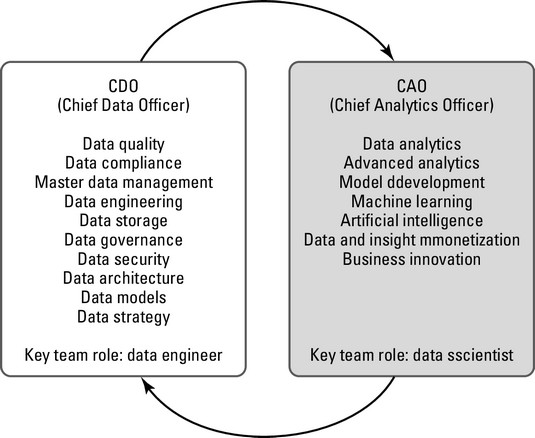
If the CDO is about data enablement, the CAO role is about how you drive insights from that data — in other words, how you make the data actionable. The CAO is much more likely to have a data science background, and the CDO, a data engineering one.
Let’s clarify that both the CDO and CAO positions are essentially carve-outs from the traditional CIO job in the IT domain. In the case of the CDO role, the CIO may well have welcomed eliminating some of these responsibilities. However, when it comes to the portion of the CIO role that is about IT cost for new data assets, the CIO can be deeply challenged by the new realities of big data. Both the CDO and CAO would need to argue for initially storing huge amounts of data, even if its value isn’t immediately evident. These aspects pose a significant but important change in mindset for the CIO role, one that probably would not have been recognized the same way without the introduction of the CDO and CAO roles.
When it comes down to the practical implementation of this role, it’s all about securing an efficient end-to-end setup and execution of the overall data science strategy across the company. Which solution can function as the most optimized setup for your company will depend on your line of business and how you’re organized. Just remember to keep these two roles working closely together, including the teams that are attached to the roles. Separation between data engineering teams and data science teams is not advisable, especially since there is a need for a strong common foundation based on these two parts in data science. The teams may have a different focus, but they need to work closely together in an iterative way to achieve the speed, flexibility, and results expected by the business stakeholders.
In cases where the CDO role is the only role in a company — where CDO and CAO responsibilities have been merged, in other words — the mandate of the CDO role is usually described in terms of the image you see below. The area referring to the business mandate refers mainly to driving areas such as:- Establishing a company-wide data science strategy
- Ensuring the adoption of a dominant data culture within the company
- Building trust and legitimizing the usage of data.
- Driving data usage for competitive advantage
- Enabling data-driven business opportunities
- Ensuring that principles for legal, security, and ethical compliance are upheld
- Establishing a data architecture
- Securing efficient data governance
- Building an infrastructure that enables explorative and experimental data science
- Promoting the continuous evolution of data science methods and techniques
- Designing principles for legal, security, and ethical aspects
- Securing efficient data and model life-cycle management
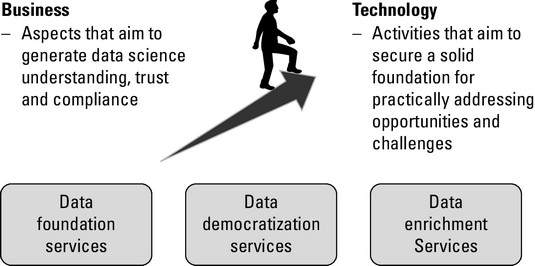
Notice that above, three distinct services a merged CDO/CAO must manage. This list gives a sense of what each service entails:
- Data foundation services includes areas such as managing data provenance and data stewardship, data architecture definition, data standards, and data governance as well as risk management and various types of compliance.
- Data democratization services refers to areas such as establishing a data-driven organizational culture through the business validation of data initiatives, making non-sensitive data available to all employees (data democratization) as well as proper evaluation of available data.
- Data enrichment services includes areas such as deriving and creating value from data through applying various analytical and machine-based methods and techniques, exploring and experimenting with data as well as ensuring a smart and efficient data lake/data pipeline setup supporting a value realization of the data science investment.
Why a CDO is needed
In addition to exploring revenue opportunities, developing acquisition strategies, and formulating customer data policies, the chief data officer is charged with explaining the strategic value of data and its important role as a business asset and revenue driver to executives, employees, and customers. Chief data officers are successful when they establish authority, secure budget and resources, and monetize their organization’s information assets.The role of the CDO is relatively new and evolving quickly, but one convenient way of looking at this role is to regard this person as the main defender and chief steward of an organization’s data assets. Organizations have a growing stake in aggregating data and using it to make better decisions. As such, the CDO is tasked with using data to automate business processes, better understand customers, develop better relationships with partners, and, ultimately, sell more products and services faster.
A number of recent analyses of market trends claim that by 2020, 50 percent of leading organizations will have CDOs with similar levels of strategy influence and authority as CIOs. CDOs can establish a leadership role by aligning their priorities with those of their organizations. To a great extent, the role is about change management. CDOs first need to define the role and manage expectations by considering the resources made available to them.Despite the recent buzz around the concept of CDOs, in practice it has proven to be difficult for them to secure anything other than moderate budgets and limited resources when reporting into existing business units, like IT. Moreover, with usually only a handful of personnel, the CDO group must operate virtually by tagging onto, and inserting themselves into, existing projects and initiatives throughout the organization. This, of course, isn’t an optimal setup when it comes to proving the value of the CDO function.
For the CDO function to truly pay off, you need to break up the silos and optimize the company structures around the data. It’s all about splitting up the scope of responsibility for your IT department so that you can separate out the data assets from the technology assets and let the CDO take ownership of the data and information part, as well as the full data science cycle when there is no CAO role appointed.
Role of the CDO
The main task of a chief data officer is to transform the company culture to one that embraces an insight- and data-driven approach. The value of this should not be underestimated. Establishing a data-first mentality pushes managers at all levels to treat data as an asset. When managers start asking for data in new ways and view data science competence as a core skill set, they will drive a new focus and priority across all levels of the company. Changing a company's cultural mindset is no easy task — it takes more than just a few workshops and a series of earnest directives from above to get the job done. The idea that one must treat data as an asset needs to be firmly anchored in the upper management layer — hence the importance of the CDO role.Let this list of common CDO mandates across various industries serve as an inspiration for what can fit in your company. A CDO can
- Establish a data-driven culture with effective data governance. As part of that project, it is also vital to gain trust the trust of the various business units so that a company-wide sense of data ownership can be established. The idea here is to foster, not hinder, the efficient use of data.
- Drive data stewardship by implementing useful data management principles and standards according to an agreed-on data strategy. It is also important to industrialize efficient data-quality management, since ensuring data quality throughout the data life-cycle requires substantial system support.
- Influence decision-making throughout the company, supported by quality data that allows for analytics and insights that can be trusted.
- Influence return on investment (ROI) through data enrichment and an improved understanding of customer needs. The idea here is to assist the business in delivering superior customer experiences by using data in all applicable ways.
- Encourage continuous data-driven innovation through experimentation and exploration of data, including making sure that the data infrastructure enables this to be done effectively and efficiently.
- Assigning business meaning to data: A CDO must make sure that data is prioritized, processed, and analyzed in the right business context in order to generate valuable and actionable insights. One aspect of this could be the timing of the insight generation: If the time it takes to generate the insight is too slow from a business usefulness perspective, the insight, rather than steering the business, would merely confirm what just happened.
- Establishing and improving data governance: The area of data governance is crucial for keeping data integrity during the life cycle of the data. It’s not just about managing access rights to the data, but very much about managing data quality and trustworthiness. As soon as manual tasks are part of data processing activities, you run the risk of introducing errors or bias into the data sets, making analysis and insights derived from the data less reliable. Automation-driven data processing is therefore a vital part of improving data governance.
- Promoting a culture of data sharing: In practice, it is the common data science function that will drive the data science activities across the company as it promotes data sharing from day to day. However, it’s also significant to have a strong spokesperson in management who enforces an understanding and acceptance of data sharing. The main focus should be to establish that you won't be able to derive value from data unless the data is used and shared. Locking in data by limiting access and usage is the wrong way to go — an open data policy within the company should be the starting point. With that in place, you can then limit access on sensitive data and still ensure that such limitations are well motivated and cannot be solved by using anonymization or other means.
- Building new revenue streams, enriching and leveraging data-as-a-service: A person in the CDO role also promotes and supports innovation related to data monetization. This is an inspiring task, but not always an easy one. Driving new business solutions that require data-driven business models and potentially completely new delivery models might inspire a lot of fear and resistance in the company and with management in general. Remember that new data monetization ideas might challenge existing business models and be seen as a threat rather than as new and promising business potential. "Be mindful and move slowly" is a good approach. Using examples from other companies or other lines of business can also prove effective in gaining trust and support from management for new data monetization ideas.
- Delivering Know Your Customer (KYC) in a real and tangible fashion: Utilizing data in such a way that it can enable data-driven sales is a proactive and efficient way to strengthen customer relationships and prove how knowledgeable the company is. However, there should be a balance here in how data is obtained and used: The last thing you want is for your customers to feel intruded on. You want the company to be perceived as proactive and forward-leaning with an innovative drive that is looking out for its customers, not as a company that invades its customers' private sphere, using their data to turn it into an advantage in negotiations. The CDO must master that balance and find a way to strategically toe that line — a line that can be quite different, depending on your business objectives and line of business.
- Fixing legacy data infrastructure issues while investing in the future of data science: This challenge is tricky to handle. You can't just switch from old legacy infrastructures (often focused on data transactions and reporting) to the new, often cloud based infrastructures focused on handling data enablement and monetization in completely new and different ways. There has to be a transition period, and during that period you have to deal with maintaining the legacy infrastructure, even when it's costly and feels like an unnecessary burden. At the same time, management is expecting fast and tangible results, based on the major investments needed. But be aware that the longer you have the two infrastructures working in parallel, the harder it is to truly get people to change their mindset and behavior toward the new data-driven approach realized through the new infrastructure investments. Neither will you see any real savings, because you need to cost-manage both the legacy environments and the new environments. Even if it proves difficult, try to drive this swap with an ambitious timeline, keeping in mind that there’s no going back.